Fundamentals of AI
In the context of the current AI revolution, several core AI technologies that challenge the existing learning paradigms need to be explored. Within different groups in the centre, several of those paradigms are being developed addressing both theoretical and practical limitations of the current ones.
Central topics within this theme in the Centre for Multimodal AI are:
- Learning from noisy data and expert knowledge: Learn with few data; learn with noisy/few/no annotations; transfer knowledge across different domains; distil knowledge of other AI systems/models; and utilise domain expertise and human knowledge.
- Adaptive and Generative AI: Continuously learn from un-curated, real-world data so as to generate synthetic data in a controllable manner and to adapt to new domains and new tasks.
- Explainable, Trustworthy, Robust and Ethical AI: Develop AI systems that can provide explanations on how and why they reach their decisions; can say how confident they are in them; are robust to attempts to manipulate them; and behave in ways that are fair and aligned with our goals and values.
- Principled Reinforcement Learning: Sample efficient methods balancing exploration and exploitation, learning forward models, Bayesian RL, benchmarking problem difficulty and agent performance.
- Causal AI: The integration of causal representation into AI systems, so as to discover and model the underlying causal mechanisms of a problem. This is achieved through structure learning algorithms or leveraging existing causal knowledge. Causal models are employed to estimate the effect of hypothetical interventions, aiding decisions by reducing or eliminating the need for conducting experiments or randomised controlled trials.
- Efficient AI: Fast, efficient and lightweight AI models with little computational and storage requirements, and thus small carbon footprint.
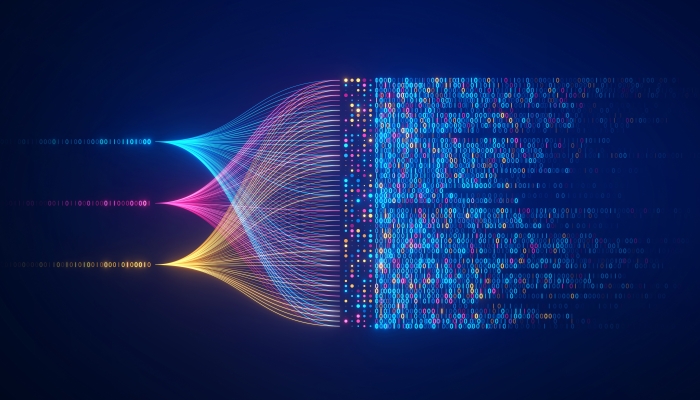